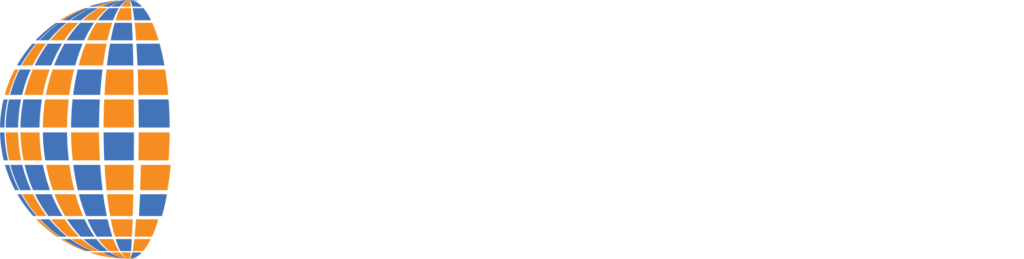
Accelerating Geospatial Machine Learning
SpaceNet delivers access to high-quality geospatial data for developers, researchers, and startups. Before SpaceNet, computer vision researchers had minimal options to obtain free, precision-labeled, and high-resolution satellite imagery. SpaceNet focuses on four open source key pillars: data, challenges, algorithms, and tools.
SpaceNet 8: Flood Detection Challenge Using Multiclass Segmentation
Each year, natural disasters such as hurricanes, tornadoes, earthquakes and floods significantly damage infrastructure and result in loss of life, property and billions of dollars. As these events become more frequent and severe, there is an increasing need to rapidly develop maps and analyze the scale of destruction to better direct resources and first responders. To help address this need, the SpaceNet 8 Flood Detection Challenge will focus on infrastructure and flood mapping related to hurricanes and heavy rains that cause route obstructions and significant damage. The goal of SpaceNet 8 is to leverage the existing repository of datasets and algorithms from SpaceNet Challenges 1-7 and apply them to a real-world disaster response scenario, expanding to multiclass feature extraction and characterization.
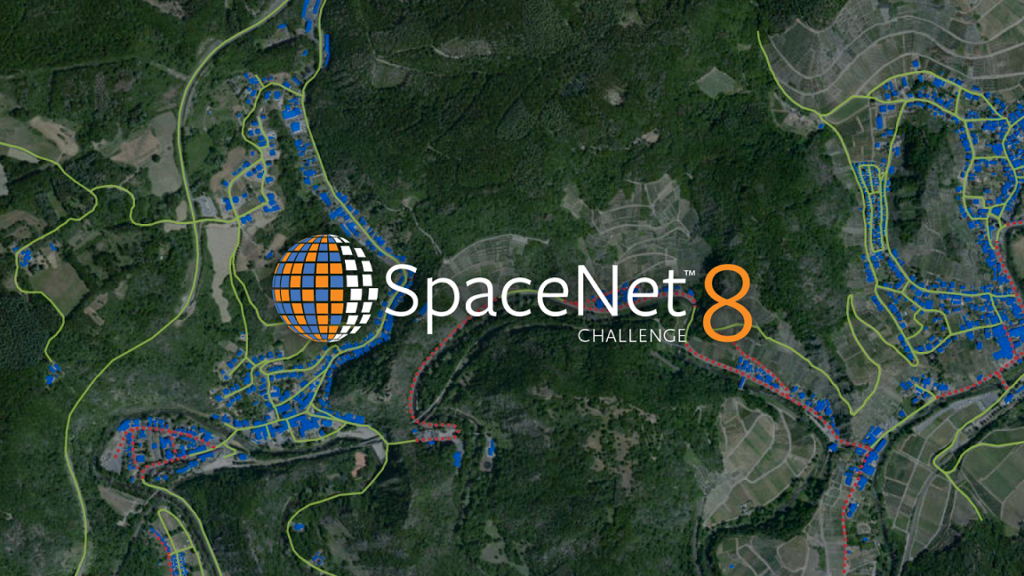
Celebrating SpaceNet
For the past five years SpaceNet has been accelerating open source, applied research in geospatial machine learning. Nine supporting Partner organizations and seven challenges later, this video highlights the key accomplishments thus far of this one-of-a-kind initiative.
Algorithm Repository
Explore algorithms used to solve SpaceNet challenges. These currently include 13 open source Building footprint and Road Network extraction problems.
Alumni Collaborators
IQT Labs’ CosmiQ Works
Co-founder & Managing Partner
Challenge Manager: SpaceNets 1-7
2016 – 2021
Capella Space
2019 – 2021
Intel AI
2018 – 2020
NGA
2016 – 2020
Planet
2019 – 2020